The Essential Guide to Bounding Boxes in Data Annotation
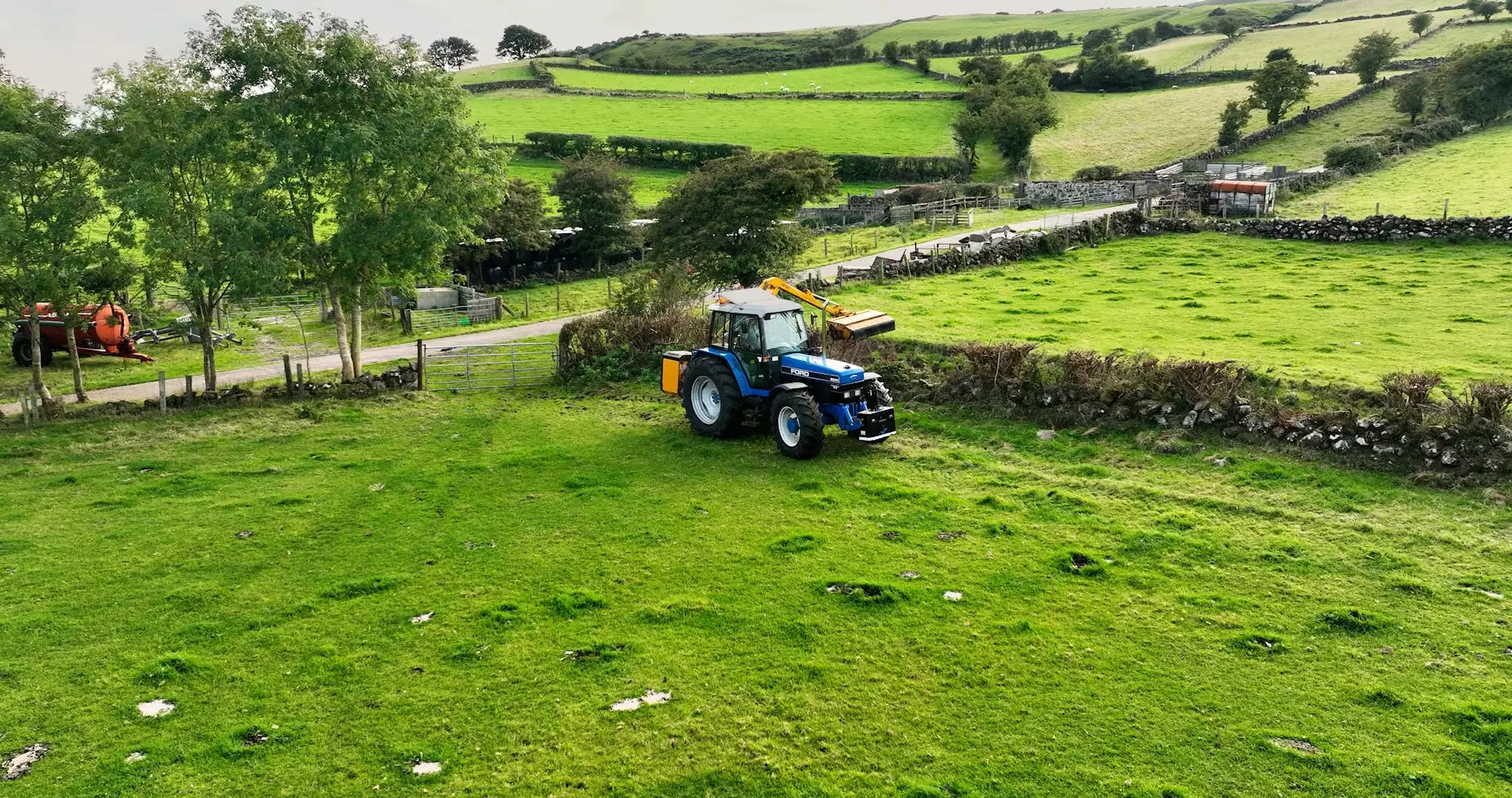
The rise of artificial intelligence (AI) and machine learning (ML) has revolutionized the way businesses operate. One of the fundamental components of training effective AI models is data annotation. In the realm of data annotation, the concept of bounding boxes has emerged as a crucial tool for providing context and accuracy to visual data. This article delves deep into the significance, applications, and methodologies surrounding bounding boxes in data annotation.
What are Bounding Boxes?
Bounding boxes are rectangular boxes that are drawn around objects within an image or video. They serve as crucial indicators in the realm of computer vision, helping algorithms identify and understand the context of the object being analyzed. These boxes can be adjusted in size and position to perfectly encapsulate the object, providing vital data that helps AI systems learn from visual inputs.
Why are Bounding Boxes Important in Data Annotation?
Understanding the importance of bounding boxes can significantly enhance the effectiveness of your AI models. Here are several reasons why they are integral to data annotation:
- Object Detection: Bounding boxes are essential for training models that need to identify and locate specific objects within images. This is particularly vital in applications such as autonomous vehicles, security surveillance, and image recognition.
- Data Clarity: By delineating objects, bounding boxes provide clarity in dataset annotations, ensuring that the data used for training is accurate and reliable.
- Annotation Efficiency: Using bounding boxes can speed up the annotation process. Annotators can quickly identify and mark the objects of interest without the need for more complex segmentation techniques.
- High-Quality Training Data: The accuracy of bounding box annotations directly affects the performance of AI models. High-quality bounding boxes lead to more effective learning and better predictions.
Applications of Bounding Boxes in Various Industries
The versatility of bounding boxes is seen across numerous sectors, showcasing their utility and importance in real-world applications. Let’s explore some of these:
1. Automotive Industry
In the automotive sector, especially with the rise of autonomous vehicles, bounding boxes play a critical role. They help in detecting pedestrians, other vehicles, road signs, and obstacles, ensuring the safety and efficiency of self-driving cars.
2. Healthcare
In medical imaging, bounding boxes are used to highlight tumors, organs, and other points of interest in MRI scans and X-rays. This assists healthcare professionals in diagnosis and treatment planning.
3. Retail and E-commerce
In the retail space, AI models utilize bounding boxes for inventory management and customer behavior analysis. They help in identifying products within images for automated checkout processes and personalized marketing strategies.
4. Agriculture
In precision agriculture, bounding boxes help in identifying crop types and assessing crop health through aerial images collected by drones. This data is crucial for optimizing farming practices and improving yields.
Methods for Creating Bounding Boxes
Creating effective bounding boxes involves various methodologies and best practices. Here are key steps to consider when annotating data:
1. Selecting the Right Tools
Choose robust data annotation platforms such as Keylabs.ai that provide intuitive interfaces for drawing bounding boxes. An ideal tool should allow for easy manipulation and adjustment of box dimensions.
2. Understanding Object Boundaries
It's essential to visualize and comprehend the edges of the object you are annotating. Proper positioning of the bounding box around the object can significantly influence the accuracy of your dataset.
3. Avoiding Overlaps
When annotating multiple objects in an image, ensure that the bounding boxes do not overlap excessively. This helps maintain clarity and prevents confusion in the dataset during training.
4. Quality Control and Review
After creating bounding boxes, implementing a quality control process is crucial. This can include peer reviews or using automated tools to check for accuracy and consistency across annotations.
Best Practices for Bounding Box Annotation
To ensure high-quality annotations, consider the following best practices:
- Consistency: Ensure that the criteria for drawing bounding boxes are consistent across all images. This includes guidelines on how tightly to draw the boxes around objects.
- Annotation Guidelines: Develop clear guidelines for annotators to follow. This might include what counts as an object, how to handle occlusions, and dealing with different object orientations.
- Iterative Refinement: Encourage an iterative approach where annotations are periodically reviewed and improved based on feedback and emerging needs.
- Invest in Training: Providing comprehensive training for annotators can significantly enhance the quality of bounding box annotations. They should understand the objectives and importance of their work.
Challenges in Using Bounding Boxes
While bounding boxes offer many advantages, there are notable challenges associated with their use:
- Accuracy Concerns: Poorly drawn or inaccurately sized bounding boxes can mislead AI models, resulting in ineffective training and subpar performance.
- Complex Object Shapes: For irregularly shaped objects, maintaining a bounding box can be challenging. The rectangular shape may not adequately capture the true dimensions of the object.
- Occlusion: In crowded scenes where objects overlap, annotators may struggle to create clear and precise bounding boxes.
Future of Bounding Boxes in Data Annotation
The field of data annotation continues to evolve, and so too does the methodology surrounding bounding boxes. The rise of advanced technologies, such as deep learning and neural networks, is leading to innovative approaches that improve how bounding boxes are utilized:
- Automated Annotation Tools: The development of AI-driven annotation tools is paving the way for more efficient and accurate bounding box creation. These tools can learn from existing annotations to suggest or automate box placement.
- Integration with Advanced AI Models: As models become more sophisticated, the need for higher precision in bounding boxes will increase. This will lead to more advanced techniques in object detection, such as using polygonal segmentation to better capture object outlines.
- User-Generated Data: Community-driven platforms for data annotation are likely to flourish, where users can contribute to labeling data, thus promoting collaboration and large-scale data generation.
Conclusion
In the rapidly advancing fields of AI and machine learning, the significance of bounding boxes in data annotation cannot be overstated. They are integral for creating accurate and reliable datasets, enabling effective training of AI models across various industries. By adopting best practices and maintaining high-quality standards in bounding box annotations, organizations can unlock the full potential of their AI initiatives. As technology continues to evolve, staying engaged with the latest trends and practices surrounding bounding boxes will ensure that businesses remain at the forefront of innovation.