The Essential Role of Labeled Image Datasets in Today's Digital Landscape
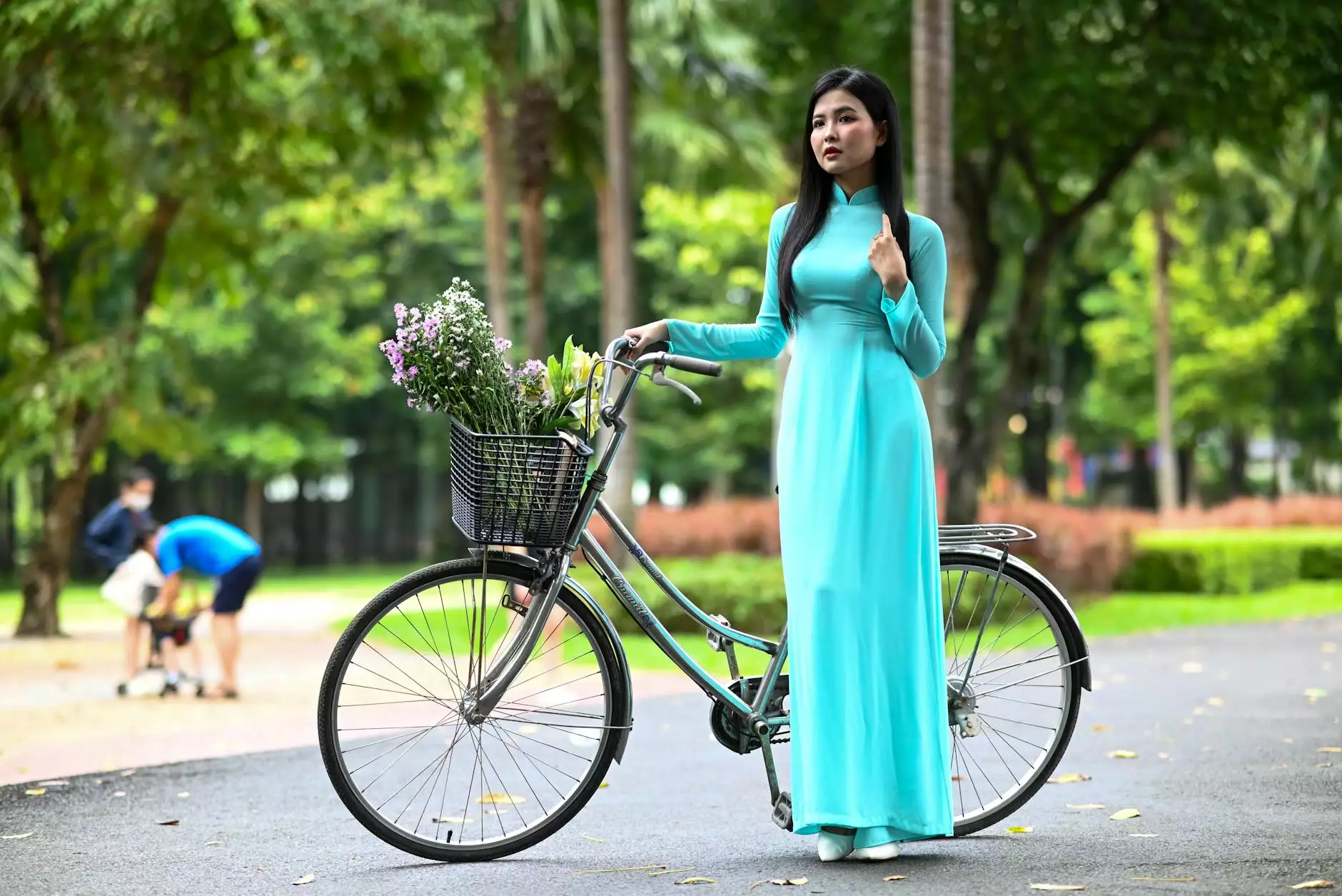
In the rapidly evolving world of artificial intelligence (AI) and machine learning (ML), labeled image datasets have become indispensable. These datasets serve as the backbone for training algorithms to recognize, classify, and interpret visual data. Whether you're in the field of autonomous vehicles, medical imaging, or e-commerce, understanding the significance of labeled image datasets is crucial for harnessing the full potential of your data.
What are Labeled Image Datasets?
A labeled image dataset refers to a collection of images that have been tagged or annotated with descriptive metadata. This metadata enables machines to understand and learn from the images presented to them. For instance, in an image dataset used for a facial recognition program, each image of a face may be labeled with names, ages, or emotional states.
Types of Labels in Image Datasets
- Classification Labels: These labels categorize images into distinct classes (e.g., dog, cat, car).
- Bounding Boxes: Used primarily in object detection tasks, bounding boxes define the region of interest in the image.
- Segmentation Masks: These provide pixel-level annotation, which is essential for applications like autonomous driving.
- Keypoint Annotations: Useful in scenarios where specific features of an object need to be identified, such as joints in human skeleton tracking.
Each type of label serves different purposes and enhances the ability of the AI models to function effectively in real-world applications.
Why Are Labeled Image Datasets Critical for Businesses?
The significance of labeled image datasets can be seen across various sectors. Here are some compelling reasons why these datasets are essential for modern businesses:
1. Enhancing Machine Learning Models
Well-annotated image datasets are vital for training robust machine learning models. High-quality datasets improve the accuracy of models, leading to more reliable predictions and decisions. Businesses that invest in quality labeled datasets often experience a significant shift in their operational efficiency.
2. Driving Innovation in Automation
As companies strive for automation, particularly in processes like inventory management or quality control, labeled image datasets pave the way for intelligent automation solutions. For instance, a retail company could use labeled image datasets to train models that automatically detect product quality on an assembly line, thus saving labor costs.
3. Facilitating Research and Development
Academic and industrial research often relies on labeled datasets to conduct experiments and validate new theories. Access to high-quality labeled image datasets enables researchers to push the boundaries of knowledge in AI and ML, thereby fostering innovation.
4. Complying with Regulatory Standards
In sectors such as healthcare, having properly labeled datasets is critical for complying with legal and regulatory standards. For instance, labeled medical images contribute to diagnostic procedures while ensuring that healthcare providers meet stringent regulatory requirements.
How to Create Labeled Image Datasets
Creating effective labeled image datasets involves several steps. Here's a comprehensive overview of the process:
1. Define the Goal
The first step is to clearly define the objective of the dataset. Different applications will require different types of labeling. Understanding what you want to achieve will guide the entire annotation process.
2. Collect Images
Gather a diverse set of images that represent the scenarios you want your AI to learn. This can include images sourced from databases, company assets, or through user submissions, depending on your needs.
3. Choose the Right Annotation Tools
Utilize specialized data annotation tools or platforms, such as those provided by KeyLabs. These tools facilitate efficient annotation through features like collaborative editing and project management capabilities.
4. Annotate the Images
Annotate the images based on the types of labels defined at the beginning. This step may involve manual labor or automated solutions, depending on the complexity and volume of the images.
5. Quality Assurance
Implement a quality assurance process to validate the accuracy of annotations. This may include peer reviews or automated checks to ensure that the dataset meets the required standards.
6. Store and Manage the Dataset
Organize the labeled images in a manner that allows for easy access and management. Proper storage solutions will help when updating or expanding your datasets in the future.
Choosing a Data Annotation Platform
Selecting the right data annotation platform is crucial for the success of your labeling projects. Here’s what you should consider:
1. Scalability
As your business grows, your data needs may evolve. A good annotation platform should allow you to scale your labeling efforts without losing efficiency.
2. User-Friendly Interface
An intuitive and easy-to-use interface can significantly reduce the learning curve for your team. Look for platforms that provide clear documentation and support.
3. Variety of Annotation Types
Ensure that the platform supports various types of annotations, including segmentation, bounding boxes, and classifications, to cater to your specific requirements.
4. Collaboration Features
If multiple stakeholders are involved, choose a platform that offers collaboration features. Real-time editing and comment functionalities can streamline communication and improve efficiency.
5. Cost-Effectiveness
Compare pricing plans and consider the ROI of investing in a particular annotation tool. Make sure it fits your budget and offers long-term value.
Case Studies: Success Stories Using Labeled Image Datasets
Numerous businesses have successfully leveraged labeled image datasets to enhance their operations. Here are some notable examples:
1. Autonomous Vehicles
Companies like Tesla and Waymo extensively use labeled image datasets to train their autonomous driving systems. By annotating diverse driving scenarios, these companies have made significant advancements in vehicle safety and navigation.
2. Healthcare Diagnostics
Healthcare organizations employ labeled images in diagnostic tools to distinguish between healthy and diseased tissues. Annotation of MRI and CT scans enables AI models to assist doctors in making informed decisions about patient care.
3. E-commerce Image Recognition
E-commerce platforms utilize labeled image datasets to develop features like visual search technology. Here, customers can upload images of products they want, and the system retrieves similar items based on visual features.
The Future of Labeled Image Datasets
As technology continues to advance, the landscape for labeled image datasets will also evolve. We are witnessing a shift towards automating the labeling process through machine learning techniques, which can significantly reduce time and effort while maintaining accuracy. Innovations like transfer learning and self-supervised learning are also paving the way for smarter processes.
Conclusion
In conclusion, labeled image datasets are a fundamental element of machine learning and AI development. They empower businesses to create intelligent systems that can analyze and interpret visual information. As the demand for AI solutions increases, investing in quality labeled datasets will be a key differentiator for businesses aimed at staying ahead in the competitive landscape. By choosing the right data annotation tools and platforms, like those offered by KeyLabs, organizations can unlock the true potential of their data and drive innovation across multiple sectors.